Project managers are always involved with data analysis and decision-making. They must be conscious of the sensitivities in data and their impact on the project. To control for this, they use sensitivity analysis to determine the sensitivity of data variables in the project outcome.
In brief, sensitivity analysis examines project scenarios under different circumstances.
This article will discuss sensitivity analysis and its benefits, compare it to scenario analysis, and provide examples of how to use it appropriately.
What is Sensitivity Analysis?
Sensitivity analysis helps determine how changes in one input affect the output. Project managers find this tool useful since it allows them to weigh the benefits and risks under different conditions.
You can see which input has the most influence on the output. Based on this information, managers can then make a better informed decision.
The sensitivity analysis can be used in the following performance domains:
- Planning performance
- The project work performance
- Delivery performance
In the sensitivity analysis process, you change one input (such as cost, time, or scope) and subsequently evaluate how the output changes. You can understand how inputs affect the outcomes by repeating the process for various inputs. After that, you may make changes to plans as needed.
Selecting the right inputs to evaluate changes while performing a sensitivity analysis is crucial. For example, in accounting, you may change the interest rate or the invested amount, but in project management, you may want to change the project’s duration or the resources needed.
It is important to understand how each input influences the outcomes. For instance, you need to know how changing a project’s duration would affect the other elements (e.g., milestones, deadlines, workloads, resource cost, etc.).
How Does Sensitivity Analysis Work?
Sensitivity analysis requires input and target variables. The fields within which you want to make changes are called input variables. The fields you want to measure the consequences of changing are the target variables.
You must develop scenarios based on your adjustments and observe changes. After that, you may use results to make choices about undertakings.
Other variables must remain constant to determine how a change in one variable may affect a result.
A “what if analysis” can show the effect of changing an input variable on the target variable.
It is important not to change more than one input variable at a time while conducting a sensitivity analysis. If you do so, you cannot identify which factors affect the result when making many changes at once. If required, you can test other variables later.
To conduct a sensitivity analysis, follow these steps.
- List the input variable that can affect the project outcome.
- Change one variable while keeping the other variables intact and note the impact of this change on the outcome.
- Repeat the above steps for all other variables.
- Rank the variable according to the severity of the impact. Keep the highest impact variable at the top and the lowest at the bottom.
Approaches for Applying Sensitivity Analysis
You can use two approaches to apply sensitivity analysis. These approaches are:
1. Direct Approach
In this method, you will directly change the numbers in a model’s assumption. For example, when using the direct technique, you may replace the growth rate with alternative values to determine the resulting revenue amounts.
For instance, the revenue calculation is as follows if your sales growth expectation is 20% annually:
(Last year’s revenue) x (1 + 20%)
2. Indirect Approach
Instead of explicitly altering the value of an assumption, the indirect technique involves inserting a % change into calculations.
For instance, if you know that the revenue formula and your revenue growth estimate is 20% annually:
(Last year’s revenue) x (1 + 20%)
You alter the formula as follows:
(Last year’s revenue) x (1 + (20% + X)), where X is a value in the sensitivity analysis area of the model.
Benefits of Sensitivity Analysis
The following are some advantages of sensitivity analysis:
#1. It Examines Many Scenarios
This approach provides probable outcomes in the event of change. Management can easily comprehend the effects and make contingency plans. It will predict the result based on the effect, which may occur when variables change.
#2. Enhanced Managerial Judgment
Sensitivity analysis provides a wide range of potential outcomes that might occur due to changes in a variable. The business will be in a much better position to make decisions after considering all available information.
#3. Effective Resource Management
Sensitivity analysis can aid in ensuring resource distribution is optimal. The business must keep a secure space. Moreover, it should enhance its resources in areas where it lags considerably behind its rivals.
#4. Highlights Areas for Improvement
Sensitivity analysis aids decision-makers in determining where they can make modifications.
#5. Provides a Higher Level of Credibility
By putting financial models to the test against a wide range of potential outcomes, sensitivity analysis increases their trustworthiness.
Sensitivity Analysis Disadvantages
- Since variables often depend on each another, it is impossible to analyze them separately. For instance, a change in selling price will result in a change in sales volume.
- The analysis is based on historical data and experiences, which might not be relevant in the future.
- Determining the highest and the lowest value depends on the decision maker’s interpretation and risk preferences. A wrong choice influences the analysis accuracy.
- It is neither a method of risk measurement nor risk mitigation. It does not result in a more transparent decision-making process. The information must be correctly interpreted.
- All factors in real life are liable to change. A simulation is a good option if you want to evaluate several variables simultaneously.
- Sensitivity analysis only reveals the consequences of changing a variable. It does not indicate the likelihood that those changes will occur.
- Sensitivity analysis will reveal various effects on the result, but it does not identify the optimal option. It just gives information on potential consequences.
Sensitivity Analysis in Different Industries
Sensitivity analysis is used in many industries. Some examples are as follows:
- Chemistry: Sensitivity analysis is used by scientists like chemists to determine measurement positions.
- Social Sciences: Econometric models may be developed using sensitivity analysis to forecast economic patterns in the future.
- Business: Sensitivity analysis is tool companies use to plan future data flow, allocate resources, and pinpoint critical assumptions.
- Meta-Analysis: Sensitivity analysis determines if constraints lead to sensitive outcomes, such as decisions that a team leader must make quickly.
- Engineering: Engineers test their designs and models through sensitivity analysis.
- Environmental: Models for assessing the effects of water purification or the global climate may be developed using sensitivity analysis.
Examples of Sensitivity Analysis
Consider the following two examples of sensitivity analysis:
Example 1
Tom is the head of the sales department of ABC corporation that sells air coolers. He knew that the sales would increase during the summer season. This year Tom wants to discover the rise in sales with increased customer traffic.
The cost of one air cooler is 700 USD. Last year during May, June, and July, the ABC company sold 200 air coolers, bringing in 140,000 USD.
After conducting a sensitivity analysis, Tom confirms that a 10% increase in customer visits during the summer months will result in a 10% increase in sales. This data helps Tom predict how much profit can be made from prioritizing additional customer visits.
Therefore, if the percentage of customer visits rises by 25% or 50%, he can expect a big boost in sales.
Example 2
John is a sales executive who wants to understand customer growth in the new resort company he has joined.
From last year’s data, he determines that when the customer base increases by 20%, sales increase by 10%. He uses sensitivity analysis and understands that if the increase in customers in the resort is 50%, total sales should increase by 25%.
Sensitivity Analysis Templates
You may forecast sales income using this sensitivity analysis table template based on input factors like traffic increase, unit pricing, and sales volume changes. In this template, we will use the indirect sensitivity analysis approach.
Template-1
Sales volume after a change in the variable = (Previous Sales volume) x (1 + (20% + X)), where X is a value in the sensitivity analysis area of the model.
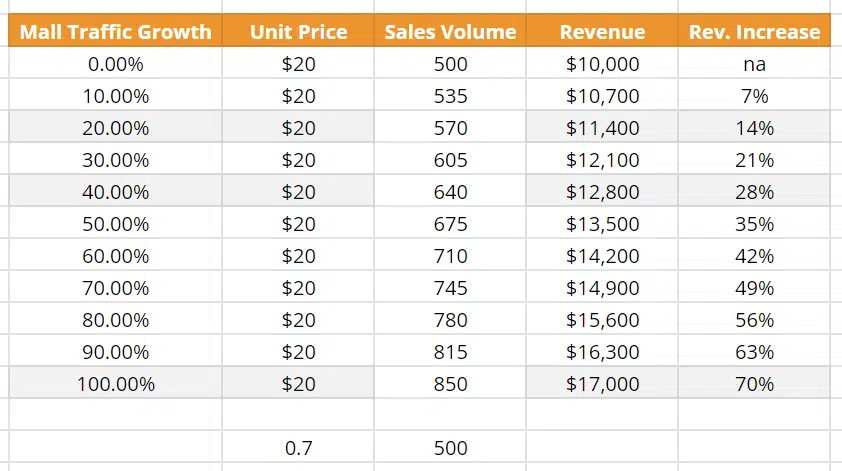
The above Sensitivity analysis template can be accessed here.
Template-2
Use the following template to display the impact of changes on business plans.
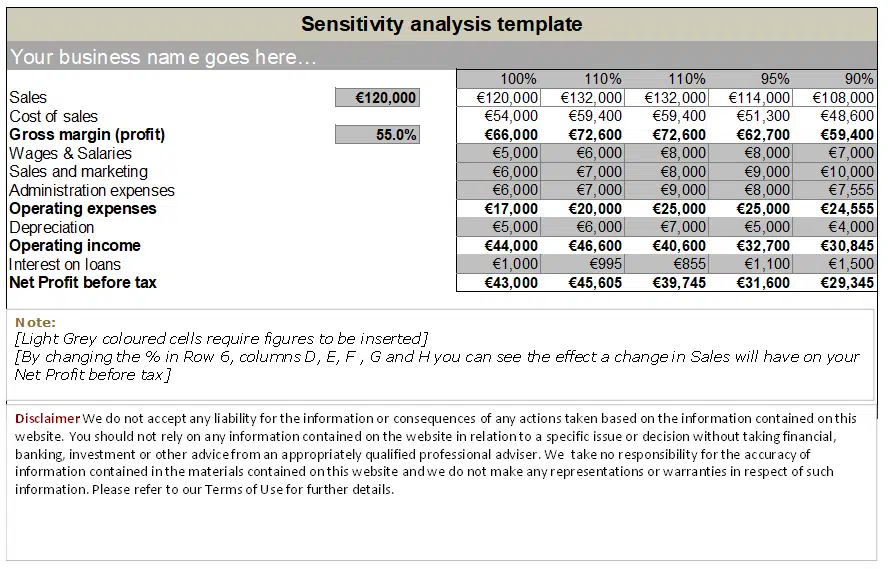
The above template is available here.
Sensitivity Analysis Techniques
The three popular sensitivity techniques are:
- Tornado Diagram
- Spider Diagram
- Monte Carlo Simulation
Sensitivity Analysis Vs Scenario Analysis
Sensitivity and scenario analysis are different techniques, although they serve the same purpose (i.e., assessing the risks or impact of changes).
In sensitivity analysis, you change one variable while keeping other variables intact and study the impact of the change on a specific outcome.
In scenario analysis, you can change the complete input scenarios and then alter all variables to align with the new scenario and study the impact of this new scenario on the outcome. Scenario analysis assesses the impact of changing all variables at the same time.
Conclusion
Sensitivity analysis is a good method to identify different outcomes by changing an input variable. You can use this analysis to find risks and opportunities and communicate them to the relevant stakeholders.
Stakeholders can see the prioritized options and then make decisions based on an objective interpretation of the data.
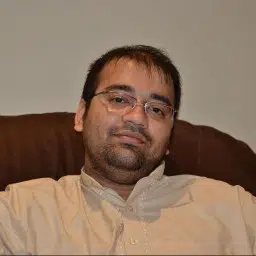
I am Mohammad Fahad Usmani, B.E. PMP, PMI-RMP. I have been blogging on project management topics since 2011. To date, thousands of professionals have passed the PMP exam using my resources.